For e-commerce businesses, managing inventory feels like a constant balancing act.
Your shelves might be overflowing with seasonal items that tie up valuable capital—while popular products sit at zero stock—leading to missed sales opportunities and frustrated customers.
When demand spikes unexpectedly, fulfilment delays strain customer relationships. Meanwhile, poor inventory predictions continue draining your resources.
Accurate demand forecasting helps solve these challenges—allowing you to optimise inventory levels, reduce costs, and meet customer expectations conistently.
This comprehensive guide walks you through everything you need to know about demand forecasting, including
- Various forecasting methods—from traditional statistical analysis to advanced AI/ML solutions
- Step-by-step strategies to select and implement the right forecasting approach
- Real-world examples and case studies of successful implementations
- Best practices for 2024
- Common pitfalls to avoid and how to overcome typical challenges
Whether you're new to forecasting or looking to improve your existing processes, this guide provides actionable insights into demand forecasting and its methods.
What is Demand Forecasting?
Demand forecasting is the practice of projecting future demand for your products.
It utilises historical indicators—such as past sales data—and current inventory patterns to create demand estimates.
The goal of forecasting is to produce precise inventory estimates that will inform your business plan and maintain a steady flow of cash into your brand.
Importance of Demand Forecasting in Modern Businesses
With accurate demand forecasting, you achieve the following benefits that help run your e-commerce business sustainably and profitably in uncertain times.

Risk Reduction: Your Safety Net
What is demand forecasting if not a way to protect your business from costly inventory mistakes, especially preventing overstock situations?
Example:
A clothing retailer using accurate forecasts ordered fewer winter coats during a mild season—saving storage costs and avoiding heavy markdowns.
Pricing Optimisation: The Smart Strategy
Forecasting enables smart pricing decisions based on predicted customer behaviour.
Example:
An electronics store adjusts laptop prices during the back-to-school season when demand peaks—and then offers discounts during slower periods to maintain sales momentum.
Customer Satisfaction: Fulfilling Customer Needs
Proper demand forecasting ensures you have the right products when customers want them—which is increasingly critical as customer loyalty patterns continue to evolve in modern retail.
Example:
A sporting goods store accurately predicted increased demand for home fitness equipment—they maintained stock levels that kept customers happy and coming back.
Improved Discount Optimisation
When products remain unsold for longer than expected, they can become a cash-in-stock liability, driving up inventory expenses and increasing the risk of obsolescence.
Eventually, these items are often sold at a discounted price, which can negatively impact your business growth.
Fortunately, demand forecasting can help you avoid this issue altogether.
Consistency: Your Business Rhythm
Demand forecasting techniques help maintain consistent inventory levels throughout the year—preparing you for seasonal shifts and demand variations.
Example:
A beauty retailer stocks more suncare products before summer and adjusts winter skincare inventory based on historical patterns and market trends.
Key Demand Forecasting Models Explained
Making sense of future demand needn't be complicated. Let's examine the most practical models brands use to predict customer demand.
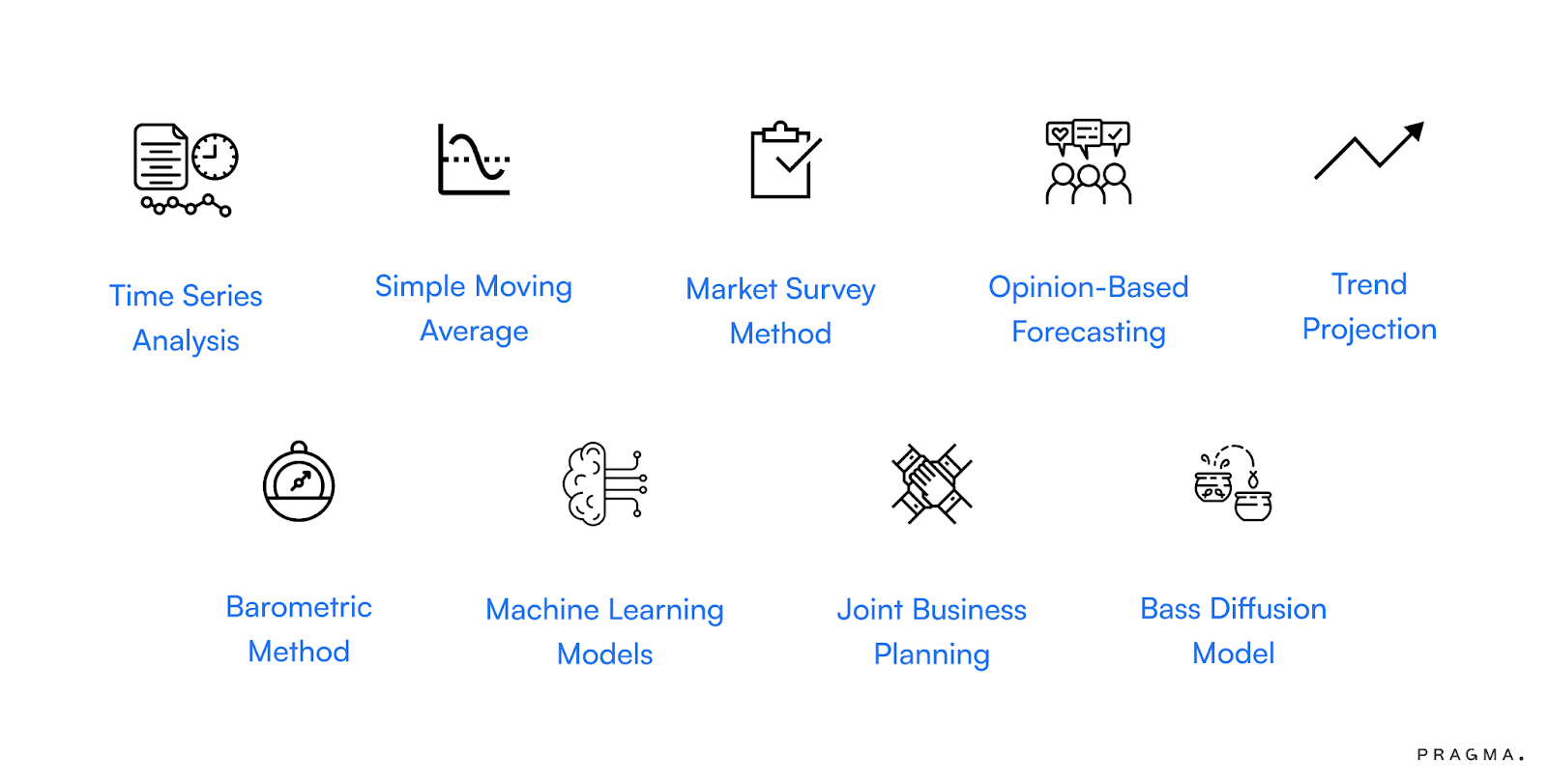
Time Series Analysis
This involves studying your sales history calendar to predict future demand.
In practice, you break down the past data points into four components and project them forward.
- Trend: The underlying pattern of growth or decline
- Cyclical patterns: Regular patterns in a data series that take
place over long periods
- Random variation (or noise): Unexplained deviation from a
predictable pattern
- Seasonal patterns: Repeatable sessions of ups and downs over
short periods
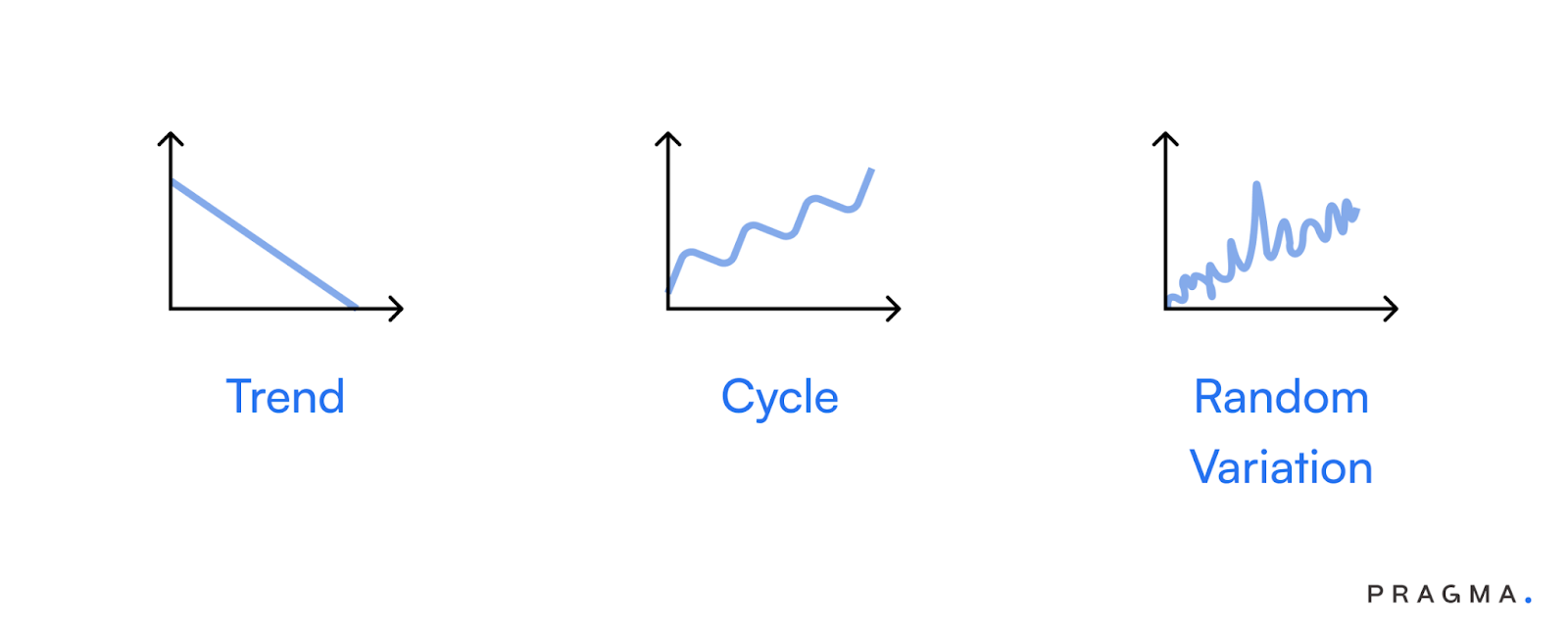
It's perfect for seasonal businesses—like retail stores preparing for holiday rushes or ice cream shops planning for summer, where effective holiday forecasting can make or break seasonal success.
Simple Moving Average
It takes the average of past demand periods.
For instance, a coffee shop might examine its last four weeks of sales to plan next week's inventory.
Market Survey Method
Sometimes the best way to know what customers want is to ask them directly via
- Customer feedback forms
- Social media polls
- Focus group discussions
- Telephone surveys
Opinion-Based Forecasting
This model brings together expert views from
- Sales teams who talk to customers daily
- Industry specialists who spot market trends
- Experienced managers who understand buying patterns
Trend Projection
In this model, you extend past patterns into the future—similar to a line on a graph.
It works particularly well for stable products with consistent growth.
Barometric Method
It involves using economic signals to predict demand.
For example, rising housing sales might signal increased demand for furniture.
Machine Learning Models
These smart-systems learn from past data to make predictions—remembering every sale and customer preference to forecast future demand.
Joint Business Planning
In this model, you work backwards from customer plans which involves
- Retailers sharing their sales targets
- Suppliers adjusting production accordingly
- Both parties agreeing on realistic numbers
Bass Diffusion Model
Perfect for new products, this model predicts how quickly customers will adopt your offering.
Consider how rapidly smartphones spread through the market—first with early adopters, then mainstream buyers.
Top Strategies for Accurate Demand Forecasting
Below are three proven strategies that make this process more accurate and reliable.
Understanding Historical Data
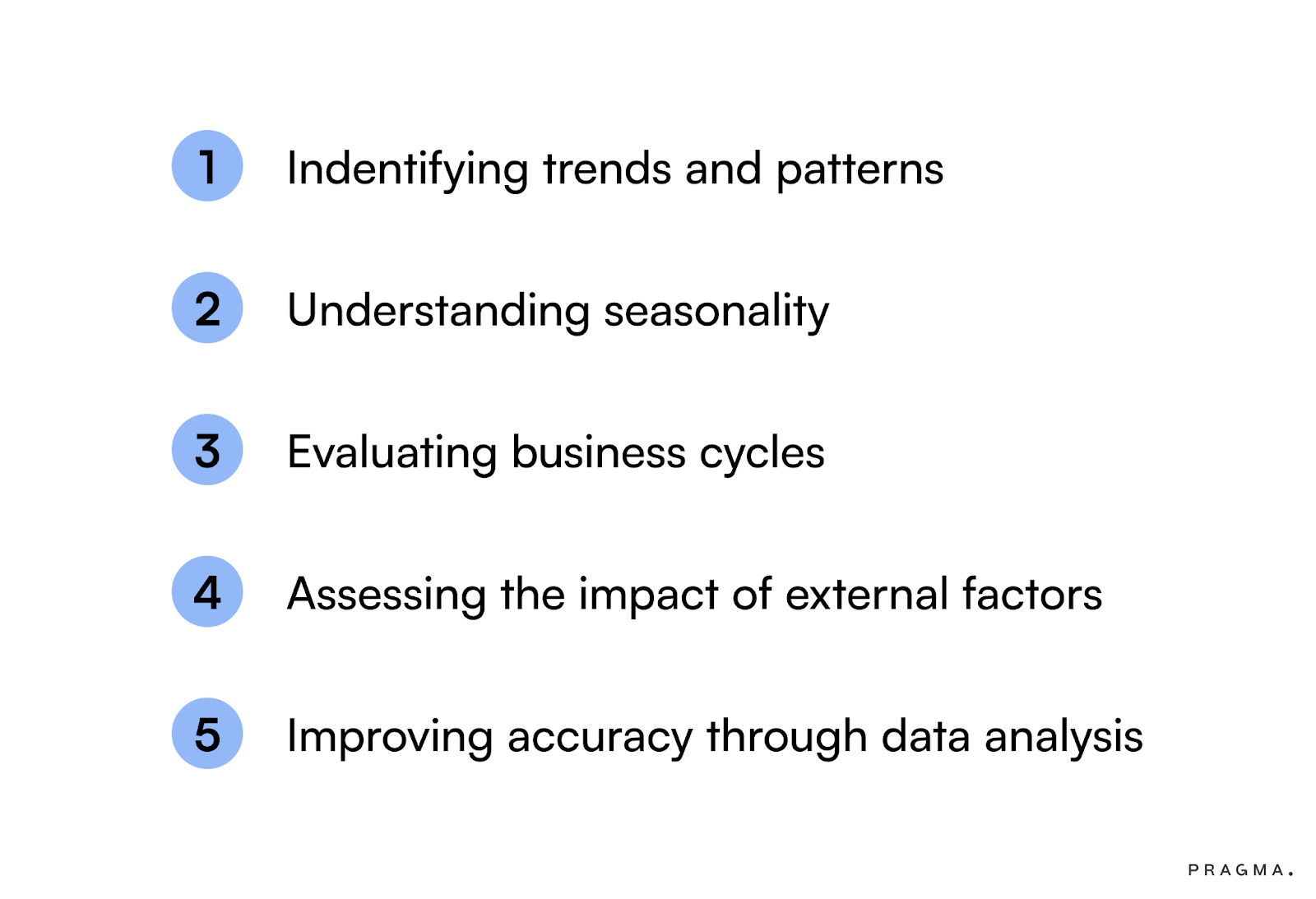
What is demand forecasting and its methods without proper data analysis?
Demand forecasting techniques begin with analysing past performance data—revealing buying patterns, peak periods, and quiet seasons.
For example, a supermarket chain studying their data notices that certain products consistently sell more during specific months—helping them optimise their inventory year-round.
Recent data reveals interesting shifts in Indian e-commerce consumer behaviour emphasising why historical data analysis is crucial.
According to Nielsen's research, online FMCG purchases now constitute 35% of all festival season purchases, up from 30% in 2019.
Indicating a clear shift in consumer comfort in buying daily essentials online.
Analysis of current customer behaviour patterns reveals distinct trends that might impact your forecasting strategies.
In terms of purchasing decisions
- 62% of online shoppers maintain strict shopping budgets
- 87% conduct thorough price research before making purchases
- Most consumers read at least three product reviews before deciding
Social influences on purchasing have also emerged as critical factors
- 75% rely heavily on recommendations from friends and family
- 72% of younger consumers trust social media influencers
- 70% make purchases driven by aspirational needs
These patterns provide valuable insights for understanding historical data and predicting future trends.
Leveraging AI and Machine Learning
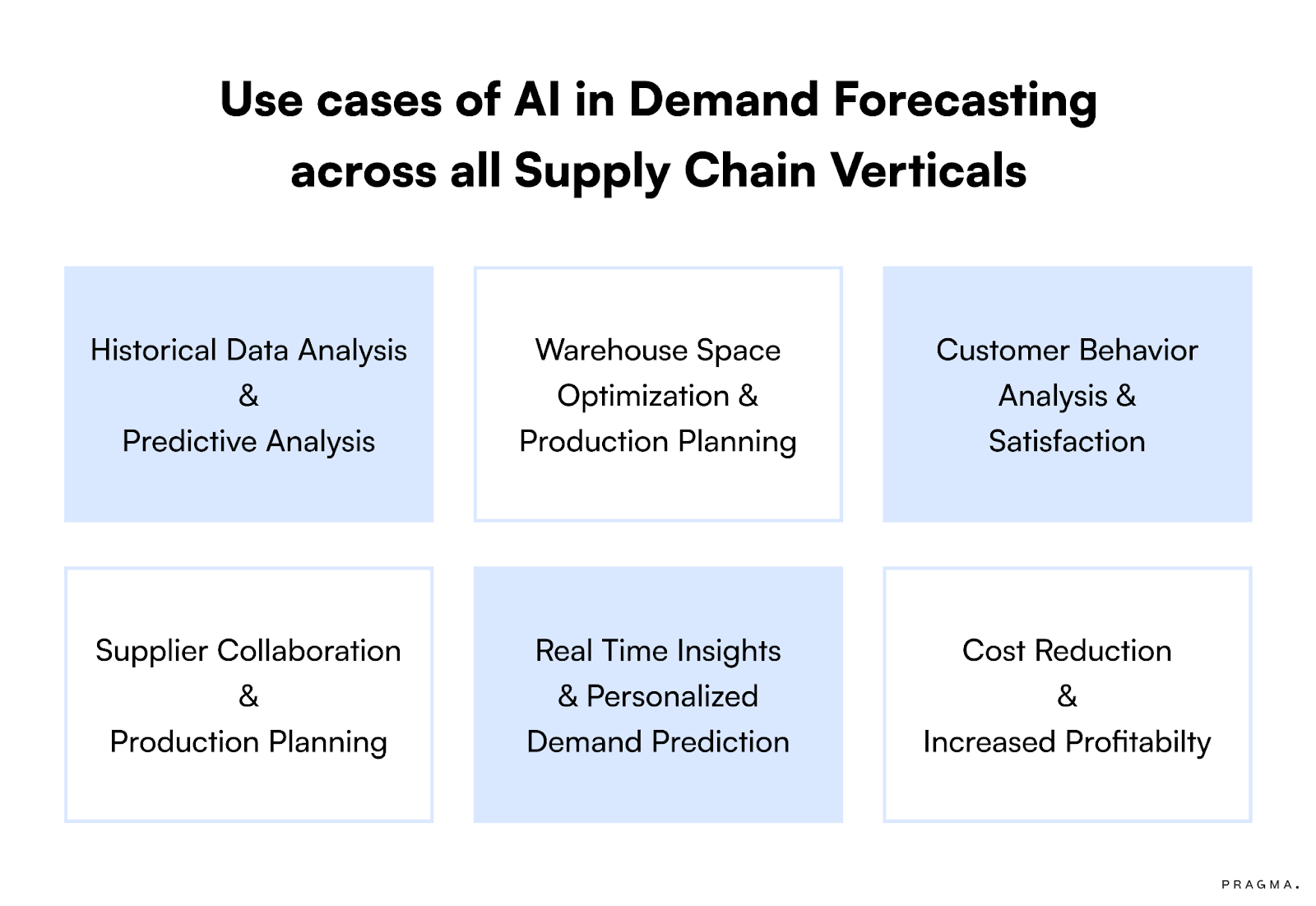
Modern demand forecasting methods now include sophisticated technologies that analyse multiple data points simultaneously. These tools examine
- Sales history
- Market conditions
- Customer behaviour
“Applying AI-driven forecasting to supply chain management, for example, can reduce errors by between 20 and 50%—and translate into a reduction in lost sales and product unavailability of up to 65%. Continuing the virtuous circle, warehousing costs can fall by 5 to 10%, and administration costs by 25 to 40%.”– Mckinsey
Consider an electronics retailer using AI tools to predict holiday season demand—the system analyses previous years' sales, current market trends, and economic factors to suggest optimal stock levels.
Addressing Seasonality and Trend
Demand forecasting techniques must account for both seasonal fluctuations and long-term market trends.
There are six common seasonality patterns you can use to define your season period and its length.
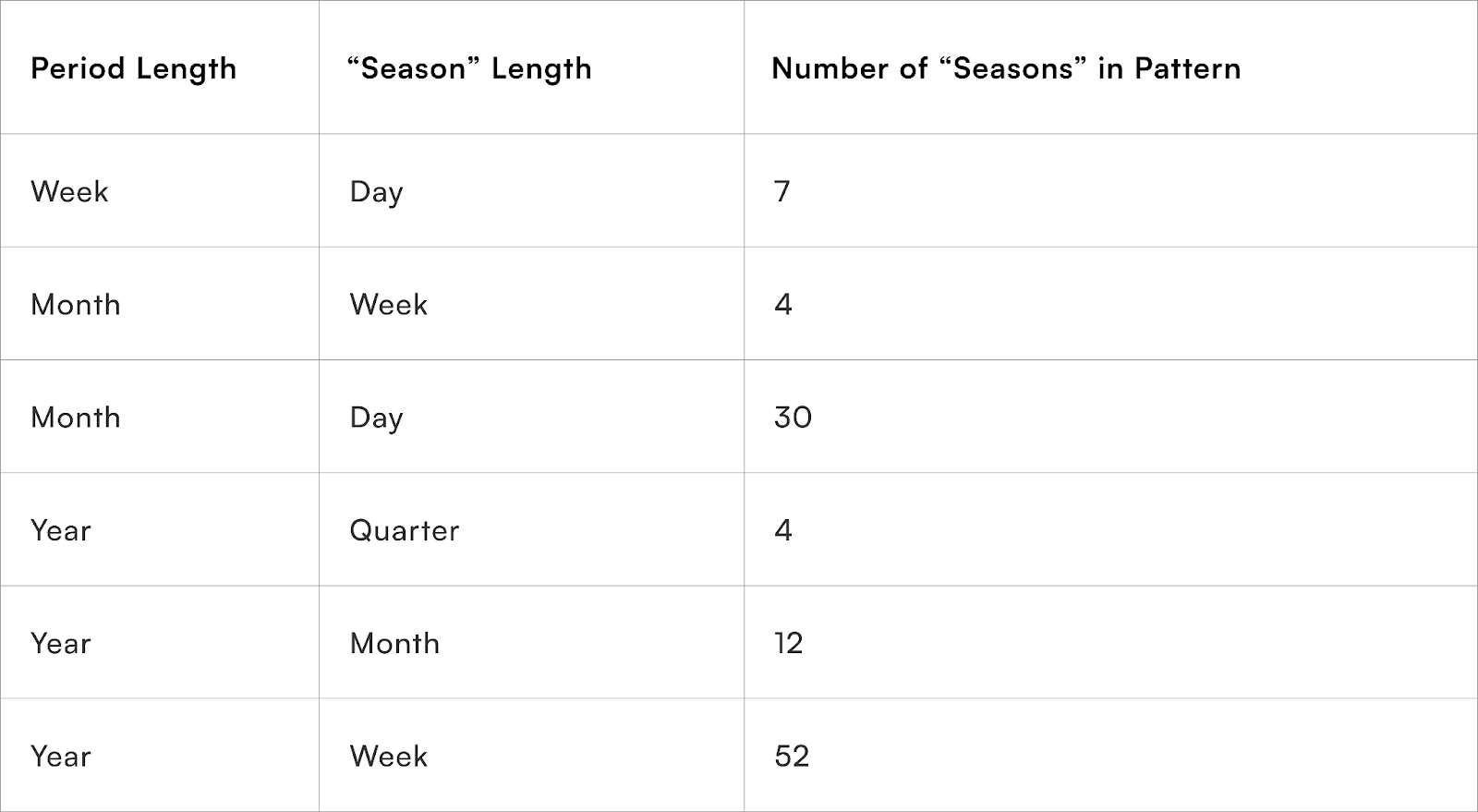
Example:
A clothing retailer shows how these patterns work together in practice
- The yearly patterns (12 months and 4 quarters) guide the major seasonal collections.
- Monthly collections transition from winter to spring, and summer to autumn, whilst quarterly planning aligns with the traditional fashion calendar.
- Weekly and daily patterns reveal shopping behaviours—weekend peaks, payday surges, and preferred shopping hours.
Special trading periods like Christmas, Black Friday sales, summer sales, and back-to-school weeks fit into the 52-week yearly pattern, requiring specific preparation and stock management.
Understanding these patterns will help you
- Maintain appropriate stock levels
- Organise staff rotation efficiently
- Plan marketing programmes effectively
- Maintain healthy profit margins
Quantitative vs Qualitative Forecasting: Which Works Best?
When it comes to demand forecasting, you must choose between two fundamental approaches—quantitative and qualitative methods.
Quantitative Methods: Data-Driven Decision Making
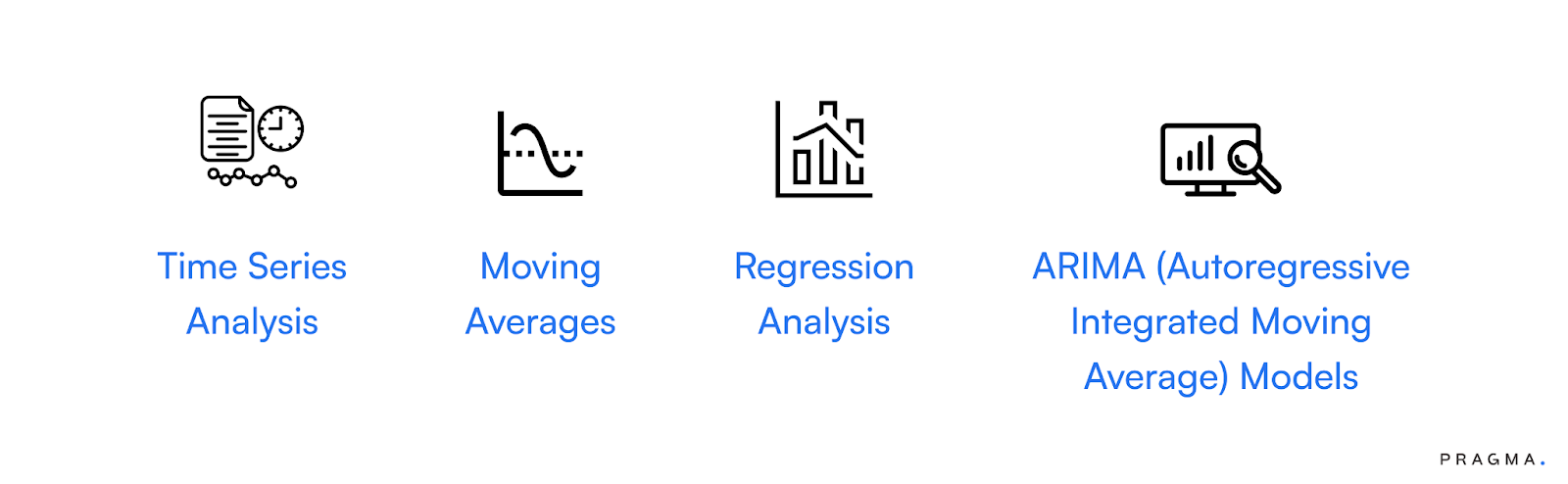
- Time Series Analysis
It examines historical data to identify patterns, trends, and seasonal fluctuations.
A retail chain might use time series analysis to predict monthly sales—accounting for seasonal peaks during holidays and slower periods during off-seasons.
- Moving Averages
This helps solve short-term fluctuations to reveal longer-term trends.
For instance, a manufacturing company might use a three-month moving average to filter out weekly variations—understanding true demand patterns for production planning.
- Regression Analysis
It helps you understand relationships between different variables affecting demand by considering multiple demand drivers simultaneously.
An e-commerce platform might analyse how factors like marketing spend, pricing, and economic indicators influence sales volumes.
- ARIMA (Autoregressive Integrated Moving Average) Models
It combines several statistical approaches to generate sophisticated forecasts—preferred for stable products with established sales histories and clear seasonal patterns.
Large retailers often use ARIMA models to automate their inventory replenishment systems.
Qualitative Methods: Human Insight and Expertise

- Expert Judgement
Expert judgement forms the foundation of qualitative forecasting—undertaking knowledge from experienced professionals who understand market dynamics, consumer behaviour, and industry trends.
A D2C company launching a new product might consult industry analysts, sales teams, and market experts to estimate initial demand.
- Delphi Method
Structures expert opinions through multiple rounds of feedback—reducing individual bias by collecting anonymous expert predictions and gradually building consensus.
Brands often use this method for long-term forecasting or when entering new markets.
- Market Research
Provides direct consumer insights through surveys, focus groups, and customer interviews—particularly valuable for new product launches or market expansions.
A food manufacturer might conduct taste tests and consumer surveys before predicting demand for a new flavour variant.
- Sales Force Feedback
Incorporates front-line insights into forecasting.
Sales teams interact directly with customers and understand local market conditions—their input helps adjust forecasts based on real-world observations and customer sentiment.
Combining Methods for Better Results
Most successful e-commerce companies use a hybrid approach to demand forecasting, where quantitative methods provide a solid baseline forecast, while qualitative insights help adjust predictions for changing market conditions.
For example, a shoe manufacturer might
- Use statistical models to analyse historical sales data and identify seasonal patterns
- Gather dealer feedback about local market conditions
- Consider economic indicators through regression analysis
- Conduct customer surveys about future purchase intentions
Choosing the Right Approach
Several factors influence the choice between quantitative and qualitative methods.
Established products with stable demand patterns benefit more from quantitative approaches. New products or volatile markets often require greater reliance on qualitative methods.
- Data Availability is Crucial
Quantitative methods need sufficient historical data to generate reliable forecasts.
When data is limited or past patterns seem irrelevant due to market changes, qualitative methods become more important.
- Forecasting Horizons Matters
Horizons is the length of time on which a forecast is based.
Short-term forecasts often rely more heavily on quantitative methods, while long-term predictions benefit from qualitative insights about market trends and industry developments.

Common Challenges in Demand Forecasting

Data Inconsistencies: The Foundation Challenge
Effective demand forecasting techniques depend heavily on data quality—it's similar to cooking, where even the best recipe fails with poor ingredients.
Key challenges include
- Fragmented sales data across multiple channels
- Misclassified product categories affecting historical patterns
- Inconsistent tracking of promotional sales vs regular sales
- Balancing warehouse management with returns processing
- Mixing seasonal and non-seasonal data incorrectly
Example
A retailer's winter coat sales are recorded at different times—store pickup, online order, or post-return period—depending on the channel. This timing mismatch makes forecasting difficult.
Unexpected Market Shifts: The Wild Card
What is demand forecasting if not an attempt to predict the unpredictable?
Like weather forecasting, external factors can quickly change the landscape
- Sudden shifts in consumer preferences
- Viral social media trends creating unexpected demand spikes
- Competitive actions—new product launches, pricing changes
- Economic fluctuations affecting buying patterns
- Supply chain disruptions impacting availability
- Changes in regulations or market policies
Understanding India's Diverse Market
The complexity of demand forecasting increases when dealing with diverse regional and cultural factors.
What works in one region may fail in another, making standardised forecasting ineffective.
Example
A clothing retailer might face different demand patterns for the same product—while lightweight cotton garments might sell year-round in southern regions, northern areas might only see seasonal demand during summer.
Festival Impact on Demand Patterns
Local festivals and cultural events present unique forecasting challenges that traditional models often fail to capture.
Example
During Diwali, demand for certain products can spike up to 300% above normal, while the same products might see minimal movement during other festivals.
Making it important to develop localised, festival-aware forecasting models.
Over-Reliance on Tools: The Technology Trap
Demand forecasting software offers powerful capabilities, but over-dependence on these tools creates new problems.
The most common pitfalls are
- Blindly trusting automated predictions without human validation
- Missing local market nuances that tools cannot capture
- Neglecting qualitative insights from sales teams
- Failing to adjust for special events or market changes
- Not considering external factors beyond historical data
A Cautionary Tale
Consider a grocery store that relied solely on forecasting software during an approaching hurricane.
The store ran out of emergency supplies, whilst competitors whose managers added extra inventory based on local experience remained well-stocked.
Additional Challenges
- Difficulties in forecasting for new products without historical data
- Managing seasonal variations across different regions
- Balancing stock levels across multiple warehouses
- Handling product transitions and phase-outs
- Accounting for changing customer behaviours in e-commerce
To ensure forecasting success, you must combine tools with human expertise, regular monitoring, and continuous adjustment against actual results.
Demand Forecasting Best Practices for 2024
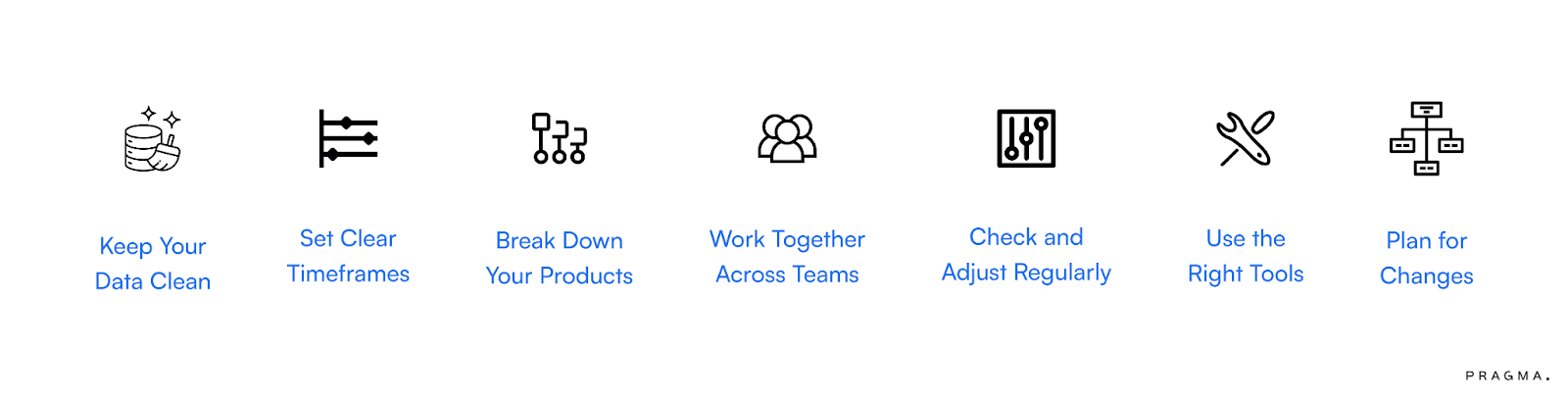
Effective demand forecasting doesn't need to be complicated.
Here are seven proven practices that will help you implement reliable demand forecasting techniques for your business.
Keep Your Data Clean
Start by ensuring accurate and up-to-date data.
Quality demand forecasting requires precise tracking of
- Actual sales
- Lost sales
- Cancelled orders
Analyse data from at least the past three years for seasonal products to spot patterns.
For example, if a customer cancels an order due to a product shortage and reorders later—count it as one demand instance to avoid inflating your numbers.
Set Clear Timeframes
Decide how far ahead your demand forecasting needs to extend.
If your suppliers need three months' notice for orders—focus on creating accurate forecasts for that period rather than longer horizons.
Some businesses need short-term predictions for the next few weeks, whilst others plan several months ahead.
For instance, fashion retailers might need longer forecasting horizons—accounting for seasonal collections and manufacturing lead times.
Break Down Your Products
Different demand forecasting techniques work better for different products.
High-volume products—like everyday essentials—typically show stable patterns, whilst speciality items might have irregular demand.
A grocery store, for example, might use simple forecasting methods for staples like bread—but need more sophisticated techniques for seasonal items like holiday decorations.
Work Together Across Teams
Successful forecasting relies on cross-department collaboration.
When the marketing team plans a promotional campaign, this information needs to reach inventory planners to adjust forecasts accordingly.
Similarly, if sales teams secure a large new contract, supply chain teams need to know to adjust their predictions.
Regular meetings between departments can prevent inventory shortages or excesses caused by miscommunication.
Check and Adjust Regularly
Monitor how your demand forecasting performs against actual sales.
If you consistently overstock certain items, your forecasting might be too optimistic.
For example, if you notice that summer product sales are lower than predicted, you can adjust future forecasts and prevent excess inventory.
Use the Right Tools
While small businesses might manage with spreadsheets, growing operations need more sophisticated demand forecasting techniques.
Modern tools can automatically detect
- Seasonal patterns
- Account for promotional impacts, and
- Adjust for market changes
A mid-sized retailer might invest in forecasting software that connects with their point-of-sale system to update predictions based on real-time sales data automatically.
Plan for Changes
Adjust your forecast when significant events occur.
Use existing store data to create initial forecasts for the new store locations.
When discontinuing products, ensure your forecasting accounts for both the phasing-out period and the new product's introduction.
It helps prevent double-stocking during product transitions or stockouts during expansion.
Future Trends in Demand Forecasting
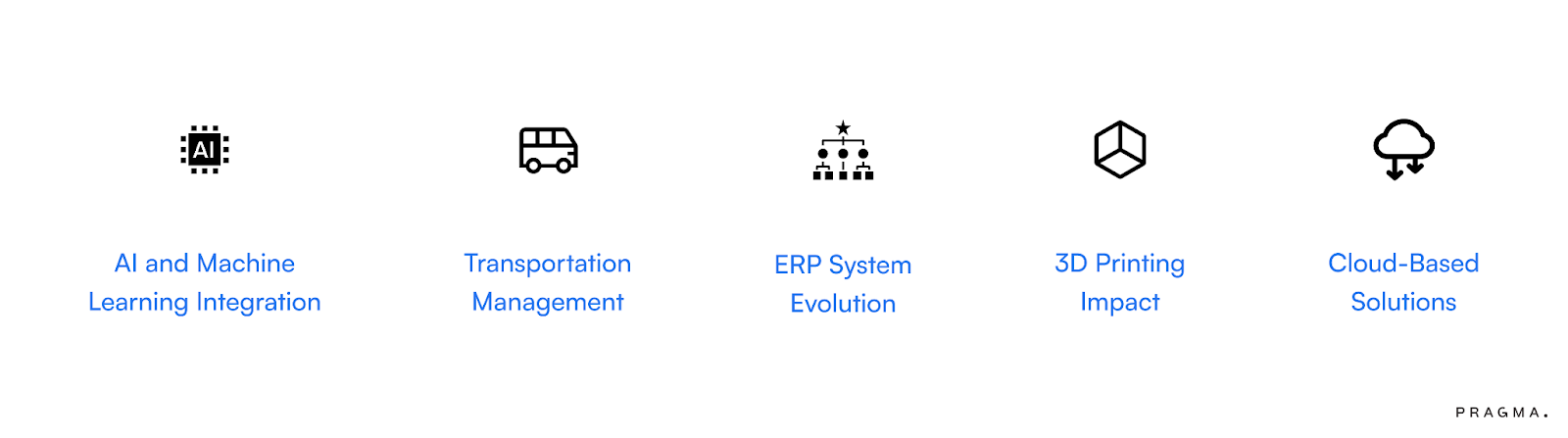
Looking ahead at the future of demand forecasting, several key technology trends are reshaping how businesses predict and manage inventory requirements.
AI and Machine Learning Integration
Artificial Intelligence and Machine Learning algorithms are becoming more sophisticated and accessible—enabling accurate demand forecasting even for smaller brands without extensive data.
Transportation Management
Real-time data integration is emerging as a significant advancement—with Transportation Management Systems (TMS) providing improved visibility throughout the supply chain.
These systems
- Track shipments precisely
- Monitor inventory movement
- Adjust forecasts accordingly
- Track temperature, location, and other vital metrics
ERP System Evolution
Integrating Enterprise Resource Planning (ERP) systems with AI capabilities helps
- Automated parameter adjustments
- Improved communication between supply chain partners
- Reduced inventory pipeline waste
- Support for sustainability initiatives
- Automatic maintenance of optimal stock levels
3D Printing Impact
3D printing technology is changing how companies handle inventory—this near-zero waste process could
- Reduce the need for large inventory stockpiles
- Enable quick response to demand changes
- Particularly benefit specialised or customised products
Cloud-Based Solutions
Small and medium-sized e-commerce companies can now access sophisticated forecasting tools through cloud-based platforms—democratising previously enterprise-only capabilities.
These solutions often include
- Built-in analytics and visualisation features
- Automated data cleaning and processing
- Real-time demand tracking
- Integration with existing business systems
- Predictive analytics for seasonal trends
- Risk assessment tools
Conclusion
Demand forecasting helps you make smarter decisions about inventory, pricing, and resource allocation.
Whilst it may seem daunting initially—the right combination of quantitative and qualitative methods can significantly improve your demand predictions.
Keep your data clean, collaborate across teams, and regularly review and adjust your forecasts.
By following the strategies and best practices outlined in this guide—you can develop more accurate forecasts to grow your e-commerce brand intelligently.
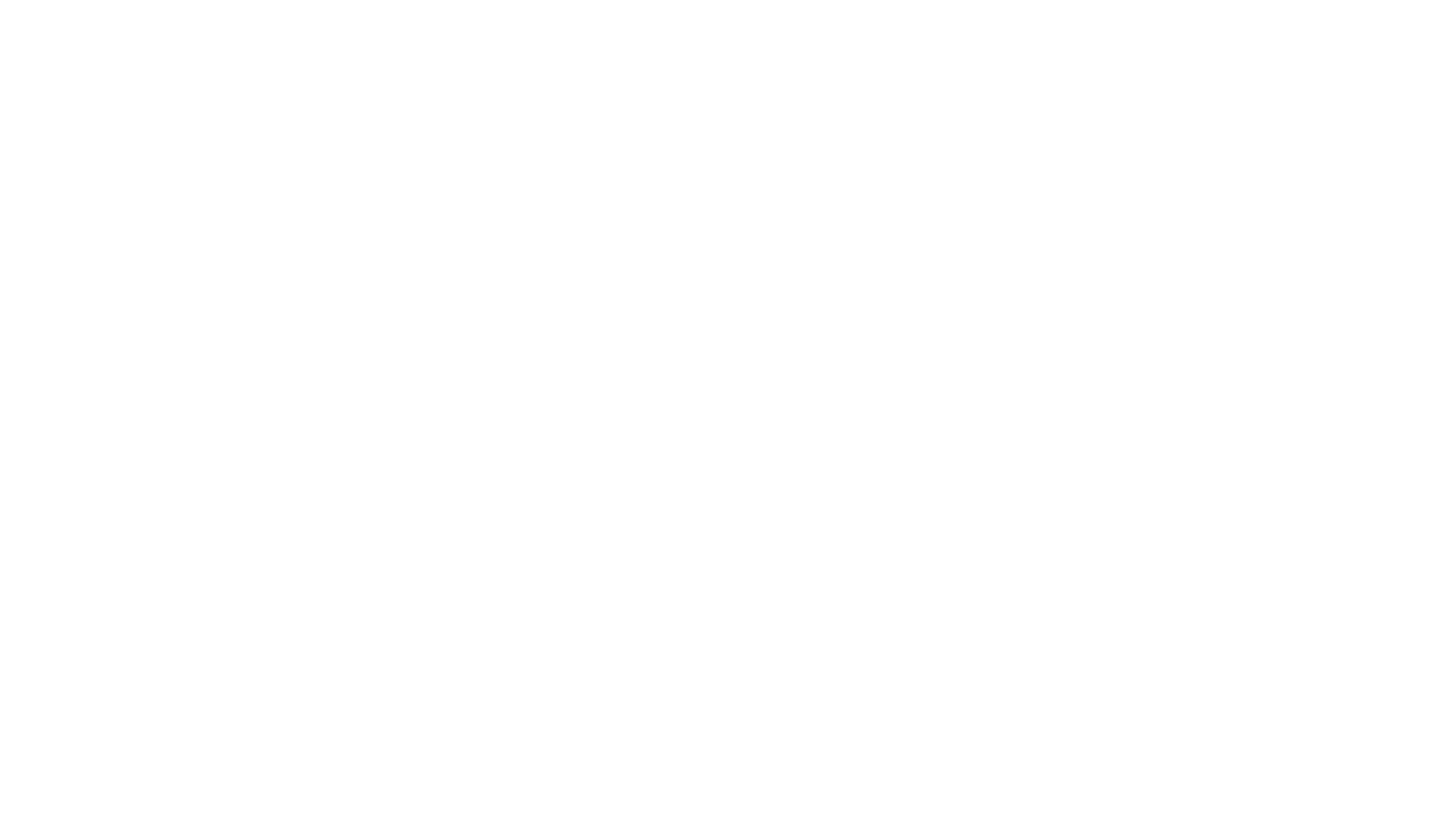
FAQ’s (Frequently Asked Questions on Demand Forecasting)
1. What are the 5 types of demand forecasting?
Five popular demand forecasting methods.
- Time Series Analysis: examines past sales patterns
- Regression Analysis: studies relationships between different sales factors
- Market Survey Method: uses direct customer feedback
- Opinion-Based Forecasting: combines expert views
- Machine Learning Models: leverages AI to analyse multiple data points simultaneously
2. What are the objectives of demand forecasting?
The main objectives are to reduce inventory risks, optimise pricing strategies, and maintain customer trust through accurate stock availability.
3. What are the characteristics of demand forecasting?
Key demand forecasting attributes include
- Historical data analysis
- Pattern recognition
- Statistical modelling
- Seasonal trend identification
- Market dynamics
- Competitor behaviour
- External factors (politics, regulations, etc.)
4. What factors affect demand forecasting in e-commerce brands?
Eight important factors that significantly affect demand forecasting
- Historical sales patterns and seasonality
- Marketing campaigns and promotional activities
- Competitor pricing and promotions
- Customer behaviour and market trends
- Economic indicators
- Product lifecycle stage
- Supply chain variables and lead times
- Online shopping patterns and website traffic
Talk to our experts for a customised solution that can maximise your sales funnel
Book a demo