Most of you might be familiar with the butterfly effect, the behaviour described by Edward Lorenz—a mathematician, meteorologist, and the father of Chaos Theory.
For those who aren’t, the simplest explanation is this: small changes can have large impacts.
And we are here to explain how things that may appear trivial, can affect complex systems and result in a large impact - i.e., The Butterfly Effect of Data on Customers!
Enabling brands with access to high-quality data is important for business success. And in today’s advanced digital world, it is easy to gather data, but not utilise it.
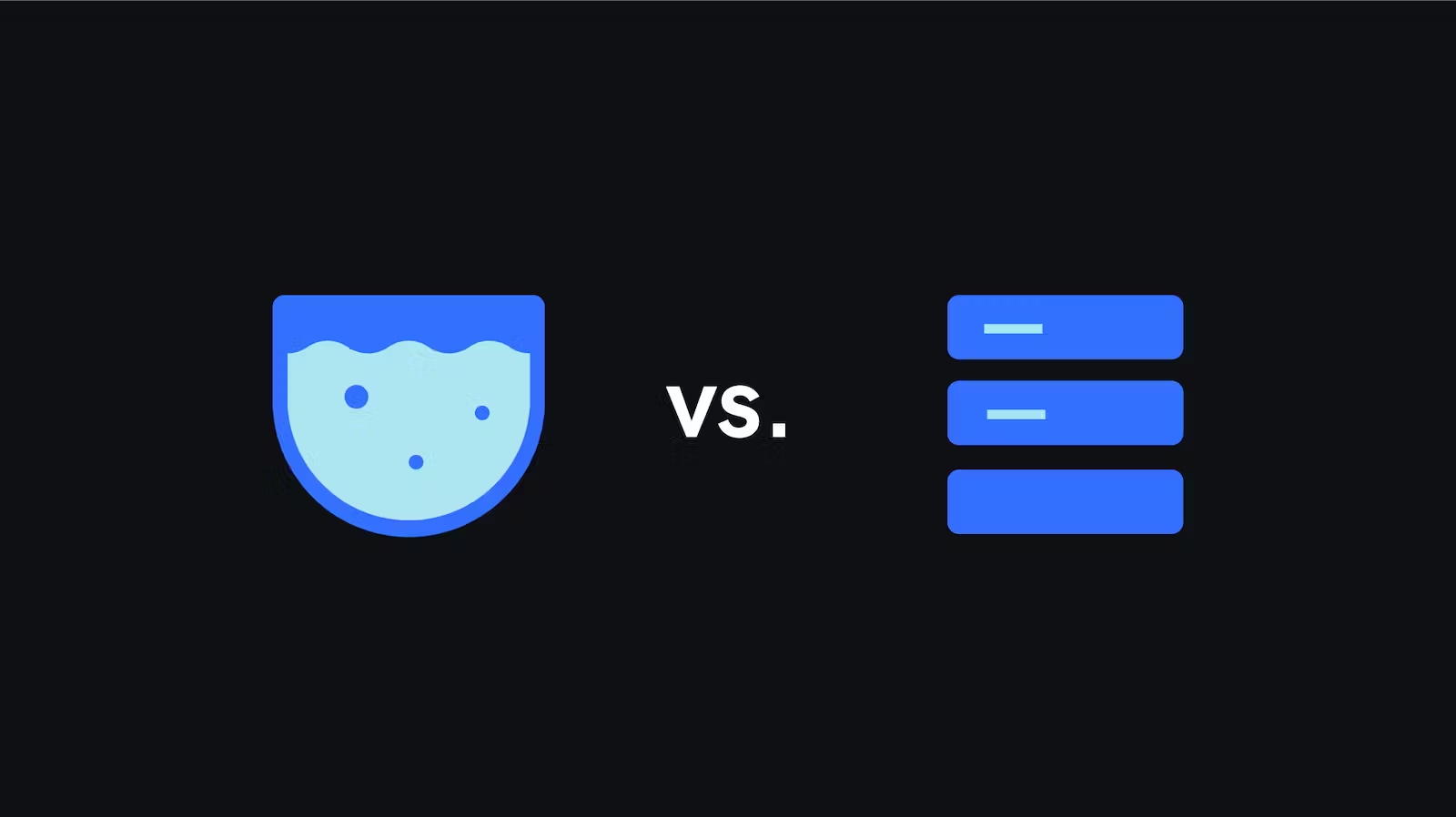
You can have a ‘Lake of Data’, but you need only a ‘Warehouse of Data’ to access what you need and whenever you need it.
Data Lakes are just a dump of large volumes of raw data, while Data Warehouses focus on structured, processed data optimised for querying and analysis.
Brands have 1 or more services in place across each stage of the customer journey to maximise customer experience (CX).
Meaning, brands can’t possibly -
- Gather the data for each of those services
- View the raw data from all those services
- And also analyse them
To conclude, if brands can get hands on someone that can provide them with a warehouse of data, (say from 450+ brands) they should capitalise on it.
Data Lake vs. Data Warehouse
Data Lake and Data Warehouse are both concepts related to managing and storing data, but they have different characteristics and purposes. Here's a comparison between the two:
Data Lake:
Definition: A data lake is a centralised repository that stores raw, unprocessed, and diverse data from various sources, such as structured, semi-structured, and unstructured data. It often uses a flat architecture and does not enforce a specific schema.
Data Storage: Data lakes store data in its original form without any predefined structure or organisation. It retains the data's native format and allows for flexible exploration and analysis.
Data Types: Data lakes can store a wide range of data types, including structured data (e.g., databases), semi-structured data (e.g., JSON, XML), unstructured data (e.g., text, images, videos), and streaming data.
Data Processing: Data lakes support data processing and analytics tools that can transform and analyse the data stored within them. The processing is typically performed on-demand, where users define the required transformations and analytics.
Schema Flexibility: Data lakes offer schema-on-read, meaning the data schema is applied at the time of data access or analysis. This allows for agility and flexibility in handling diverse and evolving data structures.
Use Cases: Data lakes are suitable for exploratory data analysis, big data analytics, machine learning, and data science projects where flexibility and agility are required.
Data Warehouse:
Definition: A data warehouse is a centralised repository that stores structured, processed, and consistent data from different sources. It follows a predefined schema and is optimised for querying and analysis.
Data Storage: Data warehouses structure and organise data into predefined schemas, such as star or snowflake schemas. It involves data modelling, extraction, transformation, and loading (ETL) processes to ensure data consistency and quality.
Data Types: Data warehouses primarily store structured data from transactional systems, such as relational databases, ERP systems, or CRM systems. It may not handle unstructured or semi-structured data efficiently.
Data Processing: Data warehouses provide pre-aggregated and pre-calculated data sets optimised for querying and analysis. They often support online analytical processing (OLAP) and business intelligence (BI) tools for complex analytics and reporting.
Schema Rigidity: Data warehouses enforce a strict schema-on-write approach, where data is transformed and loaded into predefined structures. This ensures consistency and uniformity across the data.
Use Cases: Data warehouses are commonly used for business reporting, data analysis, decision support, and generating insights from structured data.
In summary, data lakes are designed to handle large volumes of diverse, raw data with flexibility for exploration and analysis, while data warehouses focus on structured, processed data optimised for querying and analysis. The choice between a data lake and a data warehouse depends on the specific requirements, data types, and analytical needs of an organisation.
3 roles played by an ideal Data Warehouse
Various stages of customer journey requires different forms of data representation or analysis – and they are to be Data-driven, Data-informed, and Data-inspired
- Data-driven: you have the exact data you need to make a decision. If you are being data-driven, you agree with this statement, “It will tell you exactly the answer you need to know in terms of what to do next”.
Example: the historic data to optimise marketing campaigns - Data-informed: means everyone is aware of the current performance and why the product is performing the way it is in order to make optimizations to your strategies.
Example: the real-time ecommerce dashboard to keep track - Data-inspired: refers to trendspotting. This takes a few different data sources to put the story together since predicting future customer expectations is difficult to do with one data source.
Example: data from similar brands to influence optimisation
The Butterfly Effect of Data on Services
According to a survey conducted by Salesforce, 72% of consumers expect companies to understand their needs and provide personalised experiences. Furthermore, 84% of customers reported that being treated as an individual, rather than a number, influences their loyalty. This highlights the importance of D2C CRM and Omnichannel Marketing in delivering personalised product recommendations and driving customer satisfaction
Meaning, a small improvement in services across stages, will impact greatly in terms of retention and customer satisfaction.
Which is exactly why personalised product recommendations accounted for an average of 26% of e-commerce revenue.
1. Marketing & Data
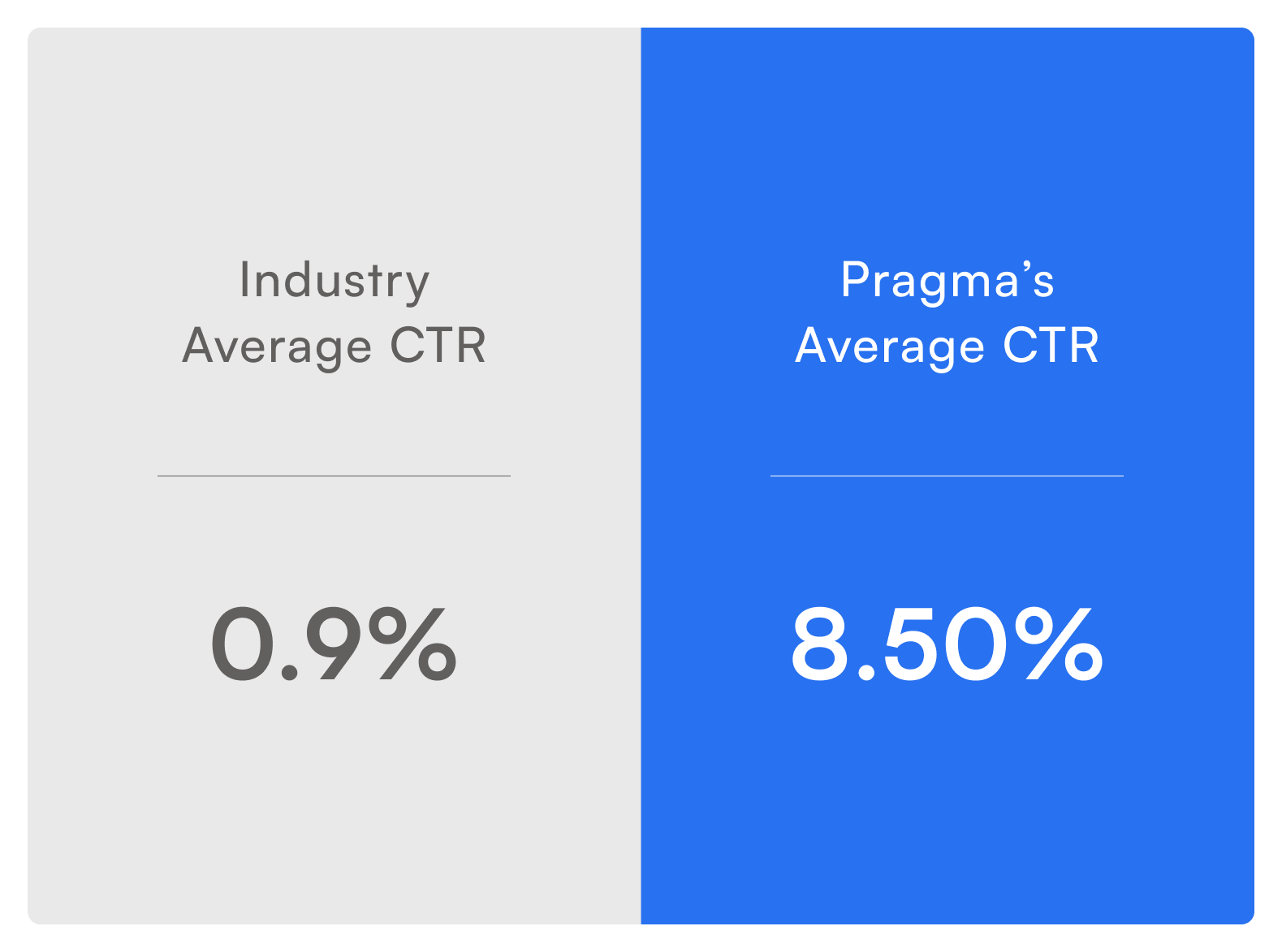
- Have access to data on individual customers from across customer journey stages, platforms, and brands
- Message individuals on channel of preference
- Understand the OR, CTR, ROAS etc of various campaigns
- Optimise campaigns based on success rate
- Increase conversions by 5-10%
📝 Fact 1: A study by McKinsey found that companies that leverage customer behaviour data to generate insights and target their messaging outperform their competitors by 85% in sales growth and more than 25% in gross margin.
📝 Fact 2: According to a study by Accenture, 91% of consumers are more likely to shop with brands that provide relevant offers and recommendations.
2. Abandoned Carts & Data
- Have access to data on where drop-offs occur
- Share real-time data with logistic partners, consumers, customer support etc. Ensure everyone is informed at all times
- Message individuals with incentives on channel of preference
- Increase abandoned cart recovery by 15-20%
📝 Fact: A study by Deloitte found that personalised loyalty rewards based on customer data can increase customer spending by 20% or more.
3. RTO & Data

- Have access to data on fraudulent users, frequent return users etc from 450+ brands
- Get another confirmation after order is placed (on customer preferred channels), when it comes to -
- Locations that cost more logistically
- Pincodes with high NDR rates
- Impulsive orders
- Disable COD option for high risk users during checkout
- Reduce RTOs by >60%
📝 Fact: Return-to-Origin orders are the highest in India because we also have the highest influx of COD order - with 16% of global RTOs coming from Indian COD orders alone.
4. Return Management & Data

- Have access to historic returns data from customers, across brands
- Understand the most frequent causes behind Returns & NDRs
- Optimise communication between: brand ↔️ logistics partner ↔️ consumer
- Reduce NDR by >20%
- Improve customer satisfaction by >15%
📝 Fact: According to a study by Aberdeen Group, companies that leverage customer analytics are 6.4 times more likely to resolve customer issues before they become problems.
The Butterfly Effect of Data in General

The butterfly effect of data on customers in the context of Direct-to-Consumer (D2C) brands in India refers to the significant impact that data can have on the overall customer experience, engagement, and relationship. Here are some key aspects to consider:
- Personalised Customer Experience: Data allows D2C brands to understand their customers' preferences, behaviours, and needs on an individual level. By leveraging customer data, brands can deliver personalised experiences, such as tailored product recommendations, customised marketing messages, and personalised customer support. This level of personalization enhances customer satisfaction and loyalty, as customers feel understood and valued.
- Supply Chain Optimization: Data can help D2C brands optimise their supply chain operations. By analysing data related to inventory levels, demand patterns, production schedules, and logistics, brands can make data-driven decisions to improve efficiency, reduce costs, and ensure timely delivery of products. This can lead to improved customer satisfaction and competitive advantage.
- Marketing Effectiveness and Efficiency: Data enables D2C brands to measure and evaluate the effectiveness of their marketing efforts. By tracking key performance indicators (KPIs) such as customer acquisition costs, conversion rates, and customer lifetime value, brands can optimise their marketing strategies and allocate resources more efficiently. Data-driven marketing approaches, including targeted advertising, social media analytics, and customer segmentation, can enhance brand visibility, reach, and impact.
- Improved Product Offerings: Data-driven insights enable D2C brands to gain a deeper understanding of customer preferences and market trends. By analysing customer data, brands can identify product gaps, emerging demands, and opportunities for innovation. This enables brands to develop and refine their product offerings to align with customer needs and expectations, leading to higher customer satisfaction and increased sales.
- Enhanced Customer Engagement: Data empowers D2C brands to engage with their customers in meaningful and relevant ways. By capturing customer interactions and preferences, brands can provide targeted promotions, loyalty rewards, and personalised content that resonates with customers. This fosters a sense of connection, builds trust, and encourages ongoing engagement, leading to long-term customer relationships through Omnichannel Marketing Campaigns and CRM.
- Seamless Customer Journey: Data enables D2C brands to create a seamless and consistent customer journey across multiple touchpoints. By integrating customer data from various channels, such as websites, mobile apps, and social media, brands can provide a unified experience. This allows customers to transition seamlessly between channels, access their purchase history, and receive personalised recommendations, resulting in a frictionless and convenient shopping experience.
- Proactive Customer Support: Data can help D2C brands anticipate and address customer needs proactively. By analysing customer data, brands can identify patterns, predict potential issues, and provide proactive customer support. For example, if a customer frequently purchases a particular product, brands can proactively notify them about relevant updates, promotions, or replenishment options, enhancing customer satisfaction and loyalty.
- Feedback and Iterative Improvement: Data allows D2C brands to gather feedback from customers and iterate their offerings based on insights. By collecting and analysing customer feedback through surveys, reviews, and social media monitoring, brands can identify areas for improvement, resolve issues promptly, and refine their products and services. This iterative approach helps brands continuously enhance their customer experience and meet evolving customer expectations.
- Operational Decision Making: Data-driven insights empower D2C brands to make informed decisions across various operational aspects. Whether it's pricing strategies, product development, market expansion, or customer service enhancements, data provides valuable inputs for strategic planning and decision-making processes. Brands can leverage Data Analytics and Omnichannel CRM tools and techniques to gain actionable intelligence and stay ahead in a competitive market.
Overall, the butterfly effect of data on customers in D2C brands in India leads to more personalised experiences, improved products, seamless journeys, proactive support, and iterative improvements. By harnessing the power of data, brands can better understand and cater to their customers, ultimately fostering stronger customer relationships and driving business growth.
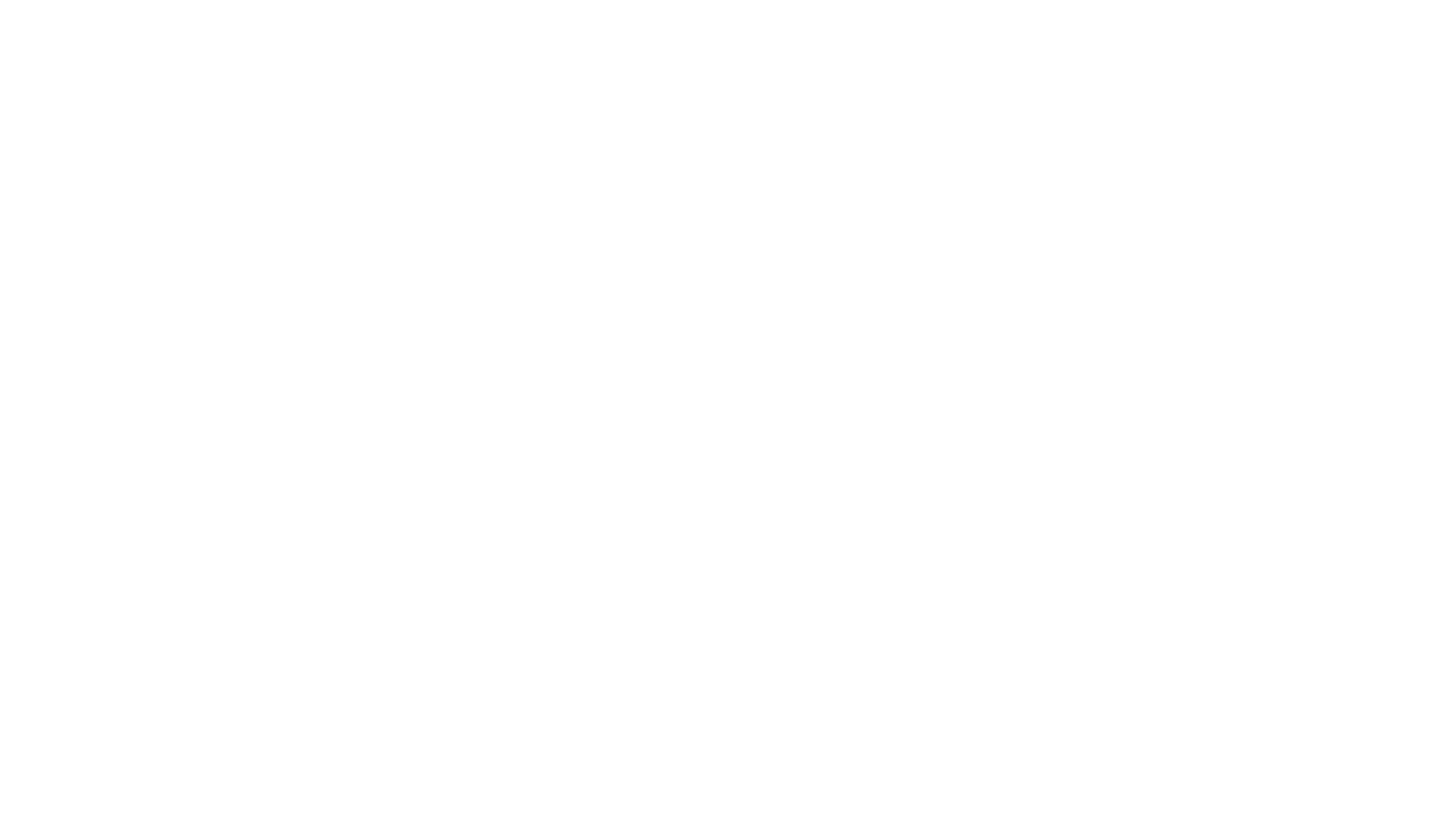
Talk to our experts for a customised solution that can maximise your sales funnel
Book a demo